Balancing AI Costs And Performance: Strategies For Running LLMs In Financial Services

Pavan Emani, SVP of Engineering at Truist Bank, is spearheading efforts to address the high costs associated with Large Language Models (LLMs) in financial services. These models, which offer capabilities such as real-time credit scoring and automated compliance, require substantial computational resources, leading to significant infrastructure expenses. Financial institutions are now focusing on strategies to optimize AI infrastructure, including fine-tuning smaller models, using retrieval-augmented generation, leveraging quantization techniques, and adopting hybrid cloud strategies to balance cost and performance effectively.
The implications of these developments are profound, as financial firms must navigate AI transformation while ensuring cost-efficiency and maintaining compliance. By focusing on cost-effective AI governance and continuous monitoring, institutions aim to prevent regulatory penalties and inefficiencies. The emergence of open-source, cost-effective models like DeepSeek-R1 is set to transform AI economics, potentially reducing LLM costs by up to 70%. This strategic approach will help financial services firms remain competitive, balancing innovation with efficiency to maximize AI's tangible business value.
RATING
The article provides a comprehensive overview of the challenges and strategies for deploying Large Language Models (LLMs) in financial services, focusing on cost and performance optimization. It is timely and relevant, addressing a critical issue in the intersection of AI and finance. However, the article's impact is limited by a lack of detailed evidence and expert opinions to substantiate its claims. While it is well-structured and clear, it could benefit from broader perspectives and more transparent sourcing. Overall, the article serves as a useful introduction to the topic but would benefit from additional depth and authoritative support to enhance its credibility and engagement potential.
RATING DETAILS
The article provides a detailed overview of the challenges and strategies related to the deployment of Large Language Models (LLMs) in financial services. It accurately identifies the high computational costs associated with LLMs, a claim supported by industry trends. However, specific claims such as the exact role of Pavan Emani and the effectiveness of strategies like fine-tuning smaller models or using Retrieval-Augmented Generation (RAG) need verification. The article mentions models like DeepSeek-R1 reducing AI costs by 50%-70%, which is a significant claim requiring concrete evidence. Overall, while the article is largely accurate, it would benefit from more direct citations to authoritative sources to substantiate its claims.
The article primarily focuses on the cost and performance balance of deploying LLMs in financial services, offering a perspective that aligns with industry efficiency and cost-effectiveness. However, it lacks a broader range of viewpoints, such as those from data scientists or IT managers who might face different challenges in implementing these technologies. The article could also benefit from discussing potential ethical concerns or the impact on jobs within the financial sector. By not addressing these aspects, the article presents a somewhat narrow view, favoring the financial and operational perspectives over others.
The article is well-structured and presents information in a logical flow, making it easy to follow. The language is clear and professional, suitable for an audience familiar with AI and financial services. However, some technical terms like 'LoRA' and 'model distillation' might require further explanation for readers less familiar with AI technology. Overall, the article communicates its points effectively, though it could benefit from a glossary or additional explanations for technical jargon.
The article does not provide specific sources or references to support its claims, which affects its credibility. While it mentions several strategies and models, such as DeepSeek-R1, it does not cite studies or expert opinions that validate these points. The absence of direct quotes or references from financial institutions or AI experts weakens the authority of the article. Including diverse and reputable sources would enhance the reliability of the information presented.
The article lacks transparency in terms of sourcing and methodology. It does not disclose how the information was gathered or if there were any potential conflicts of interest. The basis of certain claims, such as the cost savings from specific strategies, is not clearly explained. Providing more context on how conclusions were reached or citing specific studies would improve transparency and help readers understand the underlying assumptions.
Sources
YOU MAY BE INTERESTED IN
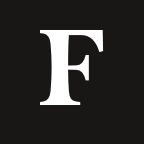
Understanding Basic Probability Is The First Step To Better Models
Score 6.8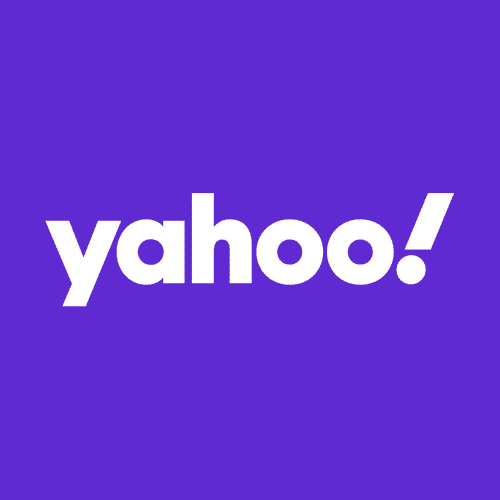
Under Trump, consumer watchdog to slash industry supervision
Score 6.8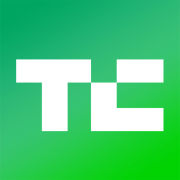
Visa-backed African unicorn Moniepoint tackles remittances. But is it late to the game?
Score 7.0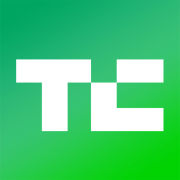
Jim Zemlin on taking a ‘portfolio approach’ to Linux Foundation projects
Score 7.0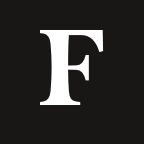