Understanding Basic Probability Is The First Step To Better Models

The story highlights the foundational role of Bayes' Theorem and conditional probability in the development of large language models (LLMs) and their applications in finance. While artificial intelligence and deep learning are often credited for recent technological advancements, it is the basic statistical principles that truly power these innovations. Bayes' Theorem helps LLMs predict the next word in a sequence and is instrumental in financial applications such as fraud detection and customer segmentation, where it updates risk assessments in real-time based on new evidence.
In the broader context, the application of Bayes' Theorem represents a significant intersection between technology and finance. Financial institutions leverage these mathematical models to enhance credit scoring, fraud detection, and customer retention strategies. This story underscores the importance of understanding basic statistical concepts, not just for data scientists, but for any executive involved in digital transformation. As AI continues to evolve, these probabilistic frameworks will remain central to driving innovation across industries.
RATING
The article provides a clear and informative overview of Bayes' Theorem and its applications in AI and finance, effectively explaining complex concepts through relatable examples. Its strength lies in its ability to make technical topics accessible to a broad audience, but it falls short in providing empirical evidence or authoritative sources to support its claims. The inclusion of promotional content somewhat detracts from the educational narrative, raising potential concerns about objectivity and transparency. While the article addresses topics of public interest and relevance, its impact is limited by its technical focus and lack of engagement with broader societal implications or ethical considerations. Overall, it serves as a useful introduction to probabilistic reasoning in modern technology but would benefit from a more balanced and well-sourced approach.
RATING DETAILS
The article accurately highlights the role of Bayes' Theorem in artificial intelligence and machine learning, emphasizing its importance in probabilistic reasoning and applications like fraud detection and language modeling. The explanation of conditional probability and its application in predicting word sequences in LLMs is consistent with established knowledge in the field. However, the article could benefit from more specific examples or empirical data to verify claims about the effectiveness of these methods in different applications, such as finance or natural language processing. The story's factual basis is strong, but some claims, like the inclusivity and predictiveness of models using conditional probabilities, would benefit from additional empirical support.
The article presents a balanced view of Bayes' Theorem's role in both AI and finance but tends to focus more on the technical aspects without delving deeply into potential limitations or criticisms of these methods. While it mentions applications in finance and language models, it could include more diverse perspectives, such as challenges in implementing these models or alternative approaches. The narrative is primarily positive, celebrating the power of statistics in modern computing, which may overlook potential biases or ethical considerations in AI applications.
The article is well-structured and uses clear language to explain complex concepts like Bayes' Theorem and conditional probability. It effectively breaks down these ideas into relatable examples, such as comparing language models to financial risk assessments. The logical flow from basic probability concepts to their application in AI and finance is coherent, making the content accessible to readers with varying levels of expertise. However, the inclusion of promotional content slightly disrupts the narrative's focus.
The article lacks explicit references to authoritative sources or studies that support its claims, which affects the perceived reliability of the information. While the explanations align with general knowledge about AI and machine learning, citing specific research papers, industry reports, or expert opinions would enhance the credibility of the claims. The absence of direct attributions makes it difficult to assess the reliability of the information presented.
The article provides a clear explanation of Bayes' Theorem and its applications but lacks transparency regarding the sources of its claims. There is no disclosure of the methodology used to derive certain assertions, such as the effectiveness of these methods in various fields. Additionally, the article includes a promotional element for an educational program, which could be perceived as a conflict of interest, yet this is not explicitly addressed or disclosed.
Sources
- https://www.almabetter.com/bytes/tutorials/artificial-intelligence/bayes-theorem-in-ai
- https://www.geeksforgeeks.org/applications-of-bayes-theorem-in-artificial-intelligence/
- https://www.geeksforgeeks.org/bayes-theorem-in-artificial-intelligence/
- https://blogs.cornell.edu/info2040/2022/11/04/bayes-theorem-in-artificial-intelligence/
- https://www.appliedaicourse.com/blog/bayes-theorem-in-machine-learning/
YOU MAY BE INTERESTED IN
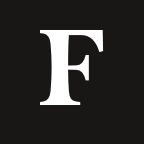
The Supply Chain Hack: AI, Data And Real-Time Insights
Score 6.2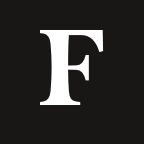
Want a Job In AI & Healthcare Tech? Here Are 5 Growth Areas Actively Hiring Right Now
Score 6.2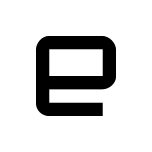
How to watch LlamaCon 2025, Meta's first generative AI developer conference
Score 7.8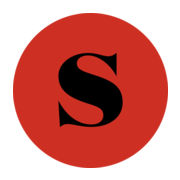
Humans think — AI, not so much. Science explains why our brains aren't just fancy computers
Score 6.6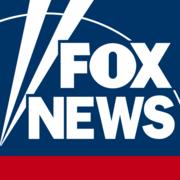