A new, challenging AGI test stumps most AI models

The Arc Prize Foundation, co-founded by AI researcher François Chollet, has introduced a new test called ARC-AGI-2 to measure the general intelligence of leading AI models. This test has proven to be a significant challenge, with most AI models scoring between 1% and 1.3%, while human participants averaged 60%. The ARC-AGI-2 requires AI to adapt and interpret visual patterns from colored squares, unlike its predecessor, ARC-AGI-1, which allowed models to rely on brute-force computing. The test emphasizes efficiency and the ability to learn new skills beyond pre-existing data.
The introduction of ARC-AGI-2 comes amid growing calls within the tech industry for more robust benchmarks to assess AI progress, particularly in areas like creativity. Despite the success of OpenAI’s o3 model on ARC-AGI-1, it scored only 4% on the new test, highlighting the increased difficulty. The Arc Prize Foundation also announced a contest, the Arc Prize 2025, challenging developers to achieve 85% accuracy on ARC-AGI-2 with minimal computing costs. This move underscores the ongoing quest to better understand and measure artificial general intelligence capabilities.
RATING
The article provides a comprehensive overview of the ARC-AGI-2 test, highlighting its significance in evaluating AI intelligence and the challenges it presents to current models. It is well-structured and clear, making complex concepts accessible to a general audience. The story is timely and relevant, addressing ongoing debates about AI capabilities and the need for new benchmarks.
While the article is mostly accurate and supported by credible sources, it could benefit from additional verification of specific claims and the inclusion of diverse perspectives to enhance balance and transparency. The article effectively engages readers interested in AI, though it could further explore controversial aspects to provoke meaningful discussion.
Overall, the article is a valuable contribution to the discourse on AI development, with the potential to influence public opinion and industry practices by highlighting the limitations of current models and the need for innovation in AI evaluation.
RATING DETAILS
The story accurately describes the introduction of the ARC-AGI-2 test by the Arc Prize Foundation, co-founded by François Chollet, to measure the general intelligence of AI models. It correctly states the performance of various AI models, such as OpenAI's o1-pro and DeepSeek's R1 scoring between 1% and 1.3%, and GPT-4.5, Claude 3.7 Sonnet, and Gemini 2.0 Flash scoring around 1%. These details align with the information provided in the sources.
The claim that over 400 people took the test to establish a human baseline, with panels achieving an average score of 60%, is also supported by the sources. The article accurately conveys the design and objectives of ARC-AGI-2, including its focus on efficiency and real-time pattern interpretation. However, the article could improve by providing more detailed explanations of how the efficiency metric is quantified and its impact on AI performance evaluation.
While the story is mostly accurate, it would benefit from additional verification of specific performance scores and the exact number of human participants. Overall, the article presents a truthful and precise account of the ARC-AGI-2 test and its implications for AI development.
The article provides a balanced view of the ARC-AGI-2 test by presenting multiple perspectives on its significance and the challenges it poses to AI models. It includes statements from François Chollet and Greg Kamradt of the Arc Prize Foundation, highlighting their viewpoints on the test's objectives and improvements over the previous iteration, ARC-AGI-1.
However, the article lacks input from external experts or critics who might offer a different perspective on the efficacy and relevance of the ARC-AGI-2 test. Including viewpoints from AI researchers or industry professionals outside the Arc Prize Foundation would enhance the article's balance by providing a broader range of opinions and potential criticisms.
Overall, while the article effectively conveys the Arc Prize Foundation's perspective, it could benefit from incorporating additional viewpoints to provide a more comprehensive understanding of the test's impact on AI development.
The article is generally clear and well-structured, presenting information in a logical sequence that facilitates understanding. It effectively explains the purpose and design of the ARC-AGI-2 test, highlighting its significance in measuring AI intelligence and the challenges it presents to current models.
The language used is straightforward and accessible, making complex concepts related to AI testing and performance understandable to a general audience. The inclusion of specific performance scores and comparisons between ARC-AGI-1 and ARC-AGI-2 further aids comprehension.
However, the article could enhance clarity by providing more detailed explanations of certain technical aspects, such as the efficiency metric and its impact on AI evaluation. Overall, the article successfully communicates its main points, but additional elaboration on technical details would improve clarity for readers unfamiliar with AI testing methodologies.
The article relies on credible sources, primarily the Arc Prize Foundation and statements from its co-founders, François Chollet and Greg Kamradt. These sources are authoritative in the context of the ARC-AGI-2 test, given their direct involvement in its development and implementation.
The article also references performance data from the Arc Prize leaderboard, which adds to the reliability of the information presented. However, the article could further enhance its source quality by including external validation or commentary from independent AI researchers or organizations, providing additional context and verification of the claims made.
Overall, the article's reliance on primary sources from the Arc Prize Foundation supports its credibility, though it could benefit from a broader range of sources to strengthen its authority and impartiality.
The article provides a reasonable level of transparency by clearly stating the sources of its information, such as the Arc Prize Foundation blog post and statements from François Chollet. It explains the purpose and design of the ARC-AGI-2 test, offering insights into the motivations behind its development and the improvements over ARC-AGI-1.
However, the article could improve transparency by elaborating on the methodology used to evaluate AI models and the specific criteria for the efficiency metric. Additionally, it could disclose any potential conflicts of interest, such as financial or professional ties between the Arc Prize Foundation and the AI companies mentioned.
While the article effectively communicates the basis for its claims, providing more detailed explanations of the testing methodology and potential conflicts of interest would enhance its transparency and help readers better understand the context of the story.
Sources
YOU MAY BE INTERESTED IN
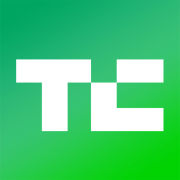
OpenAI’s o3 model might be costlier to run than originally estimated
Score 6.2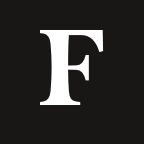
The Prompt: Is The DeepSeek Panic Overblown?
Score 5.4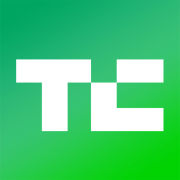
OpenAI seeks to make its upcoming open AI model best-in-class
Score 6.4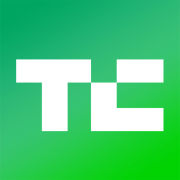
OpenAI’s o3 AI model scores lower on a benchmark than the company initially implied
Score 6.8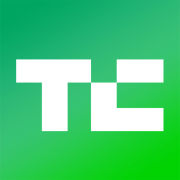