OpenAI’s o3 AI model scores lower on a benchmark than the company initially implied

A recent discrepancy between OpenAI's reported benchmark results and independent tests for its o3 AI model has sparked debates over transparency and testing practices. While OpenAI claimed that o3 could solve over 25% of the challenging FrontierMath problems, independent tests by Epoch AI found the model's performance to be around 10%. The differences are attributed to variations in testing setups, including more powerful computing resources used by OpenAI for internal testing.
The situation highlights ongoing controversies in AI benchmarking, as companies strive to promote their models by showcasing impressive results. This incident follows similar controversies in the industry, where transparency and accuracy of reported benchmark results have been questioned. As the AI landscape continues to evolve, such issues underscore the importance of skepticism and critical evaluation of AI performance claims, particularly when they come from companies with commercial interests.
RATING
The news story provides a timely and relevant examination of discrepancies in AI benchmarking, focusing on OpenAI's o3 model. It accurately presents the main claims and includes credible sources, although it could benefit from more diverse perspectives and detailed verification of the claims. The article is clear and well-structured, making it accessible to a general audience, but it could enhance engagement by including more interactive elements or visuals. Overall, the story raises important questions about transparency and reliability in the AI industry, contributing to ongoing debates and discussions.
RATING DETAILS
The news story presents several factual claims about the discrepancy in benchmark scores for OpenAI's o3 AI model. It accurately reports that OpenAI claimed a 25% success rate on FrontierMath, while Epoch AI found a 10% success rate. The story also correctly notes that the public version of o3 is less powerful than the version used for internal benchmarking. However, the article could have benefited from more detailed verification of these claims, such as specific data on the computational resources used by OpenAI and the exact versions of FrontierMath employed by both parties.
The article primarily focuses on the discrepancies between OpenAI's internal and third-party benchmark results. While it does mention Epoch AI's perspective and includes a statement from the ARC Prize Foundation, it could have provided more viewpoints, such as comments from independent AI experts or other companies in the industry. This would have offered a more balanced view of the broader implications of the benchmarking discrepancies.
The article is generally clear and well-structured, with a logical flow of information. The language is straightforward, making it easy for readers to understand the key issues involved. However, the inclusion of more technical details about the benchmarking process might improve comprehension for readers unfamiliar with AI testing practices.
The article cites credible sources such as Epoch AI and the ARC Prize Foundation, both of which are relevant and authoritative in the field of AI research. However, it lacks direct quotes or statements from OpenAI representatives, which would have strengthened the report's credibility. Including such primary sources would have provided a more comprehensive view of the situation.
The article provides some context about the testing discrepancies and mentions the potential reasons behind the differences in scores. However, it lacks detailed explanations of the methodologies used by both OpenAI and Epoch AI, which would have enhanced transparency. Additionally, the article does not disclose any potential conflicts of interest, such as financial ties between the parties involved.
Sources
YOU MAY BE INTERESTED IN
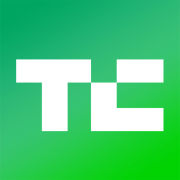
Within six years, building the leading AI data center may cost $200B
Score 5.8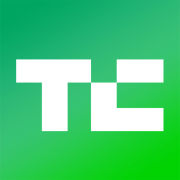
OpenAI launches program to design new ‘domain-specific’ AI benchmarks
Score 7.2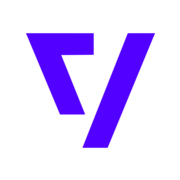
Meta got caught gaming AI benchmarks
Score 6.8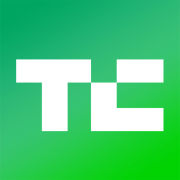
OpenAI’s o3 model might be costlier to run than originally estimated
Score 6.2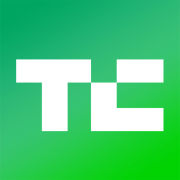