What Is High-Quality Data?

Ken Stott, CTO at Hasura, emphasizes the importance of high-quality data in driving business innovation and efficiency. He argues that implementing high-velocity feedback loops between data producers and consumers can transform data management by allowing for early issue detection and continuous improvement. This proactive approach helps organizations prevent problems at their source, enhances customer experiences, and meets regulatory compliance while reducing operational costs. Stott highlights the limitations of traditional data quality methods, which often fail at intersection points where data from different domains combines, leading to quality issues.
In addition, other experts like Weier Wan from Aizip and Kjell Carlsson from Domino Data Lab stress the need for data diversity, definiteness, and iterative quality control, particularly in the context of AI applications. They argue that traditional data cleansing and normalization methods may hinder AI's effectiveness by removing valuable signals. The story underscores the absence of standardized measures for high-quality data and the need for a nuanced approach that balances technological solutions with efficient processes. This approach should be tailored to the specific needs of AI projects and ensures that high-quality data is maintained without compromising data governance.
RATING
The article provides a comprehensive overview of data quality challenges and solutions, drawing on insights from industry experts. Its strengths include clarity, timeliness, and public interest, as it addresses relevant issues in data management and AI. However, the article's impact and engagement potential are limited by the lack of diverse perspectives, empirical evidence, and detailed examples. While the article is informative and accessible, it could benefit from a more balanced presentation of viewpoints and a deeper exploration of controversial aspects. Overall, the article serves as a useful introduction to data quality issues but could be strengthened by incorporating a broader range of sources and perspectives.
RATING DETAILS
The article presents several factual claims about data quality and management, such as the importance of high-velocity feedback loops and the roles of different organizational teams in data management. These claims are generally aligned with established data quality principles, but they lack detailed empirical evidence or case studies to fully verify their accuracy. For example, the analogy comparing data quality to products like olive oil and beef is illustrative but may oversimplify the complexities of data quality assessment. Additionally, while the article mentions the importance of feedback loops, it does not provide specific examples or studies that demonstrate their effectiveness across various organizational contexts. The mention of AI data quality issues by Kjell Carlsson is accurate in highlighting the challenges faced by traditional data quality methods, but again, lacks concrete examples or studies to substantiate the claims.
The article primarily focuses on the perspectives of industry experts like Ken Stott and Kjell Carlsson, providing a detailed view of their approaches to data quality. However, it lacks a broader range of perspectives, such as those from different industries or academic researchers, which could provide a more balanced view of the topic. The focus on specific solutions proposed by the experts might lead to an impression of favoritism towards these approaches, without exploring alternative methods or criticisms. Additionally, the article does not address potential drawbacks or limitations of the proposed solutions, which could provide a more balanced understanding of the complexities involved in data quality management.
The article is generally clear and well-structured, with a logical flow that guides the reader through the discussion of data quality and management. The language is accessible, and the use of analogies, such as comparing data quality to products like olive oil and beef, helps to illustrate complex concepts in a relatable way. However, some sections could benefit from more detailed explanations, particularly when discussing technical aspects like feedback loops and data governance. Despite these minor issues, the overall tone is neutral, and the information is presented in a way that is easy for readers to understand.
The article cites credible industry figures like Ken Stott and Kjell Carlsson, who are recognized experts in the field of data management and AI strategy. Their insights lend authority to the discussion on data quality. However, the article does not reference academic studies or independent research, which could enhance the credibility and reliability of the claims made. The reliance on industry experts may introduce a bias towards the solutions they advocate, and without diverse sources, the article may not fully capture the breadth of opinions and evidence available on the topic.
The article provides some context for the claims made by explaining the roles of data management teams and the significance of feedback loops. However, it lacks transparency in terms of the methodology behind the experts' claims and the potential conflicts of interest that might influence their perspectives. The article does not disclose whether the experts have a vested interest in promoting the solutions they discuss, such as through their affiliations with specific companies. Additionally, the absence of detailed explanations or citations for the claims made limits the reader's ability to assess the basis for these claims independently.
Sources
- https://www.alation.com/blog/what-is-data-quality-why-is-it-important/
- https://www.collibra.com/blog/the-6-dimensions-of-data-quality
- https://www.datafold.com/data-quality-guide/what-is-data-quality
- https://www.clearpointstrategy.com/blog/data-quality-metrics
- https://www.sbctc.edu/resources/documents/colleges-staff/commissions-councils/dgc/data-quality-deminsions.pdf
YOU MAY BE INTERESTED IN
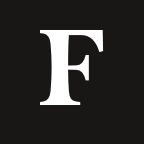
From Popcorn To Pop-Ups: The Bold New Era Of Retail And Film Collaborations
Score 6.0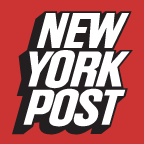
Google’s Waymo self-driving robotaxis could be put on sale for people looking to own the vehicle
Score 7.4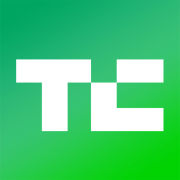
Startups Weekly: Tech IPOs and deals proceed, but price matters
Score 6.0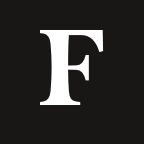
If OpenAI Buys Chrome, AI May Rule The Browser Wars
Score 7.2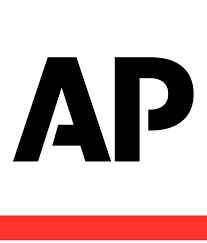