This Decentralized AI Could Revolutionize Drug Development

Rowan Labs and decentralized AI subnet Macrocosmos have joined forces to revolutionize drug discovery by leveraging AI neural networks trained on quantum mechanics. This collaboration utilizes Rowan's Egret-1, a machine-learned neural network capable of simulating organic chemistry with atomic precision. By combining Rowan's high-accuracy synthetic data generation with Macrocosmos' decentralized compute layer, the partnership aims to drastically reduce the cost and time required to discover new therapeutic compounds and treatments. This approach promises to accelerate the development of drugs, potentially shortening the drug discovery timeline by years and making cures for rare diseases more accessible.
The significance of this partnership extends beyond human health, impacting areas such as carbon capture, manufacturing, and environmental clean-up. Rowan Labs' open-source approach and commitment to transparency in model performance benchmarks enhance the credibility of their synthetic data, which is claimed to be more accurate than traditional physical experiments. The collaboration not only highlights the potential of decentralized science but also demonstrates the scalability and efficiency of decentralized AI networks like Bittensor. As the teams work towards publishing a peer-reviewed paper on their progress, the implications for science and technology are vast, suggesting a future where decentralized AI can incentivize and drive advancements across various scientific fields.
RATING
The article provides an informative overview of the partnership between Rowan Labs and Macrocosmos, highlighting the potential of decentralized AI in drug discovery. It effectively conveys the promise of faster and cheaper drug development, which is of significant public interest. However, the article could benefit from a more balanced perspective by including external viewpoints and addressing potential challenges or limitations. The reliance on statements from involved parties and the need for further verification of some claims affect the overall accuracy and source quality. Despite these limitations, the article remains engaging and timely, with the potential to influence public opinion on the role of AI in healthcare.
RATING DETAILS
The article presents several factual claims that are generally supported by available information, such as the partnership between Rowan Labs and Macrocosmos, and the release of Egret-1. However, some claims, like the speed and cost advantages of decentralized AI and the accuracy of synthetic data, require further verification. The mention of public benchmarks adds credibility, but detailed verification of these claims is needed for a higher accuracy score.
The article primarily focuses on the positive aspects of the Rowan Labs and Macrocosmos partnership without presenting potential downsides or challenges. It lacks perspectives from external experts or critics who might provide a more balanced view. The narrative leans towards highlighting the benefits of decentralized AI in drug discovery, which could suggest an imbalance in perspective representation.
The article is generally clear, with a logical flow and structured presentation of information. Technical terms are explained in simpler language, aiding comprehension. However, some sections could benefit from additional clarification, particularly regarding the technical processes involved in synthetic data generation and its applications.
The article relies heavily on statements from Rowan Labs and Macrocosmos representatives, which may introduce bias. While these sources are authoritative regarding their projects, the lack of independent expert opinions or third-party validation affects the overall source quality. Including a wider range of sources could enhance credibility and reliability.
The article provides some transparency by detailing the methodology behind the AI models and the open-source nature of the projects. However, it could improve by disclosing potential conflicts of interest and providing more context about the decentralized AI protocol Bittensor. Clearer explanations of the technical aspects and their implications would enhance transparency.
Sources
- https://www.rowansci.com/blog/can-ai-accelerate-scientific-research
- https://www.rowansci.com/blog/partnering-with-macrocosmos
- https://aidrivendrugdevelopment.com/events/ai-drug-discovery-development-summit
- https://hub-xchange.com/xchanges/ai-drug-discovery-xchange-boston-2025
- https://www.labiotech.eu/best-biotech/ai-drug-discovery-companies/
YOU MAY BE INTERESTED IN
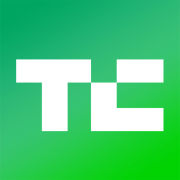
FutureHouse previews an AI tool for ‘data-driven’ biology discovery
Score 6.4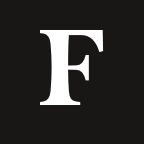
Big Pharma Is Investing Billions In AI— And The Value Is Finally Starting To Show
Score 6.0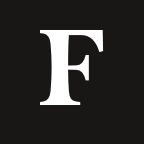
What Exactly Is Quantum Computing? And Why Is It Important For Healthcare?
Score 6.8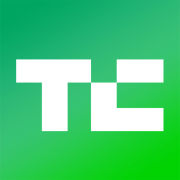
Charlie Javice trial becomes a master class in hubris for both sides
Score 5.8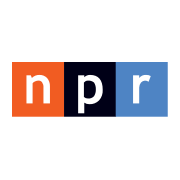