The Rise Of Retrieval-Augmented Generation

Retrieval-augmented generation (RAG) is revolutionizing the AI landscape by enhancing the precision and reliability of AI models. Co-founded by Punnam Raju Manthena of Tekskills Inc., RAG utilizes a technique that retrieves relevant documents to augment AI-generated content. Its applications span various domains such as sales, human resources, healthcare, and finance. Notably, an e-commerce giant reported a 25% increase in customer participation and a 15% boost in sales after implementing RAG for product customization and marketing.
Despite its transformative potential, RAG faces challenges such as biases in datasets, retrieval inaccuracies, and scalability issues. Ensuring data accuracy and adhering to privacy laws are critical for reliable performance. Organizations are encouraged to use diverse and current datasets while improving data storage and retrieval methods. As RAG continues to evolve, it promises to further leverage AI capabilities, offering significant benefits across multiple sectors, although careful planning is needed to mitigate its limitations.
RATING
The article provides a comprehensive overview of retrieval-augmented generation (RAG), highlighting its potential applications and challenges across various industries. It is timely and relevant, given the ongoing advancements in AI technologies and their increasing integration into business processes. The clarity and readability of the article make it accessible to a general audience, although it could benefit from more explicit sourcing and transparency to enhance its credibility.
While the article presents a balanced view of the benefits and challenges of RAG, it leans slightly towards emphasizing the positive aspects. Incorporating more detailed discussions of potential negative impacts or ethical considerations could provide a more nuanced perspective. Additionally, the inclusion of authoritative sources and expert opinions would strengthen the article's impact and influence on public and industry perspectives.
Overall, the article succeeds in engaging readers with its exploration of RAG, though it could further enhance its engagement and controversy potential by delving deeper into contentious issues and providing a platform for diverse viewpoints.
RATING DETAILS
The story provides a generally accurate depiction of retrieval-augmented generation (RAG) and its applications. The definition and process of RAG are described in alignment with existing literature, which confirms its role in combining information retrieval with generative AI to enhance precision and dependability. The story accurately outlines various applications of RAG, such as in content creation, chatbots, and human resources.
However, the article includes specific claims, such as the 25% increase in customer participation and 15% increase in sales for an e-commerce giant, which lack direct verification from cited sources. This suggests a need for more robust evidence to support these claims. Additionally, while the challenges of RAG are discussed, such as biases in datasets and scalability issues, these are consistent with known limitations of the technology.
Overall, the story is factually sound but could benefit from more explicit sourcing or citations, especially for specific case studies mentioned. The accuracy of the article is bolstered by its consistency with general knowledge on RAG, though it could be strengthened by direct references to studies or expert opinions.
The article provides a balanced view of the potential benefits and challenges associated with RAG. It highlights both the positive applications of RAG across various industries and the potential pitfalls, such as biases and scalability issues. This dual perspective helps present a more nuanced understanding of the technology.
However, the article leans slightly towards emphasizing the positive aspects and potential of RAG, with phrases like 'a great future' and 'pluses seem to far outweigh the minuses.' While it acknowledges challenges, these are not explored in as much depth as the benefits, which could lead to an impression of slight bias towards the positive impacts.
The balance could be improved by providing more detailed examples or expert opinions on the potential negative impacts or limitations of RAG, thus ensuring a more comprehensive view of the technology's impact.
The article is generally clear and well-structured, providing a logical flow of information from the definition and process of RAG to its applications and challenges. The language used is accessible and avoids overly technical jargon, making it suitable for a general audience interested in AI developments.
The tone is neutral and informative, contributing to the article's clarity. However, some sections could benefit from more detailed explanations or examples, particularly when discussing the challenges associated with RAG. This would help enhance understanding for readers who may not be familiar with the technical intricacies of AI technologies.
Overall, the article succeeds in conveying complex information in a comprehensible manner, though it could be improved by providing more in-depth explanations or illustrative examples of the concepts discussed.
The article lacks explicit citations or references to authoritative sources, which affects the assessment of source quality. While it mentions sources like 'Rag About It' and 'STX Next,' these are not well-known or widely recognized authorities in the field of AI or RAG.
The absence of direct attributions to reputable organizations or experts in AI research limits the ability to fully assess the reliability and credibility of the information presented. Additionally, the lack of direct quotes or references from industry leaders or academic studies further impacts the perceived quality of sources.
To improve source quality, the article could incorporate insights from established AI researchers, academic journals, or industry reports that provide a more authoritative basis for the claims made about RAG.
The article provides a general overview of RAG but lacks transparency in terms of sourcing and methodology. It does not clearly indicate where specific claims, such as the success metrics for the e-commerce giant, originate from, nor does it provide a methodology for how these metrics were obtained.
There is also a lack of disclosure regarding potential conflicts of interest or affiliations that might influence the portrayal of RAG. For instance, the mention of 'Forbes Technology Council' at the end of the article is not clearly linked to the content discussed, leaving readers uncertain about any potential bias.
Improving transparency would involve providing clearer attributions for data and claims, as well as disclosing any potential conflicts of interest or affiliations that could impact the article's impartiality.
Sources
- https://en.wikipedia.org/wiki/Retrieval-augmented_generation
- https://www.superannotate.com/blog/rag-explained
- https://research.ibm.com/blog/retrieval-augmented-generation-RAG
- https://www.splunk.com/en_us/blog/learn/retrieval-augmented-generation-rag.html
- https://cloud.google.com/use-cases/retrieval-augmented-generation
YOU MAY BE INTERESTED IN
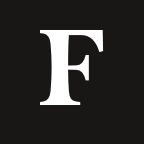
From Popcorn To Pop-Ups: The Bold New Era Of Retail And Film Collaborations
Score 6.0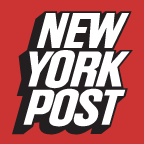
Google’s Waymo self-driving robotaxis could be put on sale for people looking to own the vehicle
Score 7.4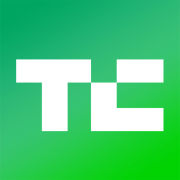
Startups Weekly: Tech IPOs and deals proceed, but price matters
Score 6.0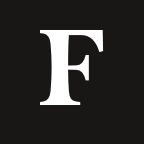
If OpenAI Buys Chrome, AI May Rule The Browser Wars
Score 7.2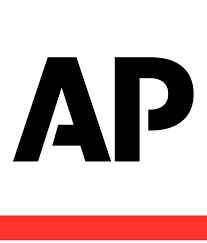