The Gaping Hole In Today’s AI Capabilities

Recent developments in AI have introduced reasoning models based on inference-time compute, bringing us closer to Artificial General Intelligence (AGI). However, a major limitation remains: the inability of AI systems to learn continuously like humans. Current AI models are static post-training, unable to incorporate new information dynamically without retraining, a process that is time-consuming and expensive. This shortcoming is being addressed by emerging approaches like perpetual learning, which is gaining traction, with startups Writer and Sakana leading the charge. Writer's self-evolving models and Sakana's Transformer2 are pioneering solutions that allow AI systems to adapt and learn in real-time, promising more personalized and efficient AI interactions.
The implications of these advancements are significant, as they could create a new type of competitive advantage in AI applications by enabling models to become more personalized with use. Continual learning could unlock new capabilities and market opportunities, making AI products stickier and more tailored to individual user needs. This shift promises to redefine AI's potential, moving towards systems that can learn and adapt like living intelligence, fundamentally transforming both consumer and enterprise settings.
RATING
The article provides a comprehensive overview of the current state and future potential of AI, particularly focusing on the challenges of continuous learning and the emerging solutions. It effectively captures the excitement surrounding AI advancements and presents an optimistic view of the future, supported by insights from industry leaders.
However, the article could benefit from a more balanced perspective, incorporating critical viewpoints and addressing potential ethical concerns. While it is timely and relevant, the lack of direct citations and reliance on potentially biased sources may impact its credibility. Overall, the article is engaging and informative, offering valuable insights into the future trajectory of AI development.
RATING DETAILS
The article accurately describes the current state of AI, particularly regarding the limitations of AI systems and the emerging solutions for continual learning. It correctly identifies the lack of continuous learning as a significant challenge in AI development, supported by the notion that AI models need retraining to incorporate new information. The claims about emerging solutions like Writer's self-evolving models and Sakana's Transformer2 align with ongoing research and developments in AI, indicating a high level of factual accuracy.
However, some claims, such as the predictions about achieving AGI within a few years, require further verification as they are speculative and based on current trends rather than concrete evidence. Additionally, while the article provides a comprehensive overview of the challenges and potential solutions in AI, some technical details about the new methodologies might need further backing from peer-reviewed studies or industry reports to enhance verifiability.
The article presents a balanced view of the challenges and advancements in AI, highlighting both the limitations of current systems and the potential of emerging technologies. It includes perspectives from industry leaders like Sam Altman and Dario Amodei, providing insights into the optimism surrounding AI's future.
However, the article could improve by including more critical viewpoints or skepticism regarding the feasibility and timeline of achieving AGI and the implementation of continual learning. By not addressing potential drawbacks or ethical concerns associated with these advancements, the article leans slightly towards an overly optimistic narrative.
The article is generally well-structured and uses clear language to explain complex AI concepts, making it accessible to a broad audience. It effectively breaks down the limitations of current AI systems and the potential of continual learning, using examples and analogies to aid understanding.
However, the article occasionally delves into technical jargon without sufficient explanation for lay readers, which could hinder comprehension. Additionally, the flow could be improved by organizing the discussion of solutions and challenges more logically, ensuring a smoother narrative progression.
The article references statements from reputable figures in the AI industry, such as Sam Altman and Dario Amodei, which lends credibility to its claims. However, it lacks direct citations or links to primary sources, such as research papers or official statements, which would enhance the reliability and authority of the information presented.
Furthermore, the article mentions specific companies and their technologies, like Writer and Sakana, without providing detailed evidence or third-party validation of their claims. This reliance on potentially biased sources, given the author's affiliation with Radical Ventures, could impact the impartiality of the reporting.
The article discloses the author's conflict of interest, noting their partnership at Radical Ventures, which invests in Writer. This transparency is commendable, although it is mentioned only at the end, after discussing Writer's innovations extensively.
The article could improve transparency by providing more context on the methodologies and data supporting the claims about continual learning and the progress of AI technologies. A clearer explanation of the basis for predictions and technical claims would help readers assess the article's impartiality and credibility.
Sources
- https://magazine.sebastianraschka.com/p/state-of-llm-reasoning-and-inference-scaling
- https://www.infoq.com/news/2025/01/openai-inference-time/
- https://openai.com/index/trading-inference-time-compute-for-adversarial-robustness/
- https://blogs.nvidia.com/blog/ai-scaling-laws/
- https://cloudedjudgement.substack.com/p/bonus-clouded-judgement-inference
YOU MAY BE INTERESTED IN
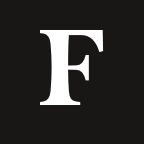
TED Reveals From OpenAI, Google, Meta Heads To Court, Selfie With Myself
Score 5.8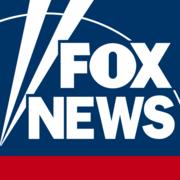
Fox News AI Newsletter: 'Battlestar Galactica' is 'even more relevant now,' star says
Score 6.8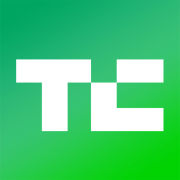
DeepMind’s 145-page paper on AGI safety may not convince skeptics
Score 7.2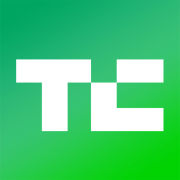
OpenAI adopts rival Anthropic’s standard for connecting AI models to data
Score 7.6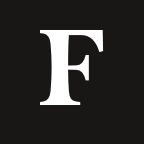