The AI Healthcare Paradox: Why Breaking Data Silos Is Key To Generalizable, Trustworthy Models

Sanjay Juneja, M.D., Co-Founder of TensorBlack Inc., highlights a critical issue in AI-driven healthcare models: the limited scope of training data. Many AI models are trained on data from isolated institutions, leading to highly specialized but narrow solutions that may not perform well in broader, diverse contexts. This poses the risk of biases that undermine the reliability and adoption of AI in healthcare. To address this, Juneja advocates for a more collaborative approach to data sharing, encompassing diverse geographic and demographic sources to enhance the generalizability and fairness of AI models.
The implications of failing to diversify AI training data are significant. Without an inclusive data strategy, AI solutions could exacerbate healthcare disparities, reduce physician trust, and face increased regulatory scrutiny. Federated learning and responsible data-sharing frameworks are proposed as solutions to balance privacy concerns while improving model diversity. Governments and regulatory bodies are urged to incentivize such collaborations, making AI's promise of standardized, improved healthcare a reality. The story underscores the need for AI models to undergo rigorous external validation and bias audits to ensure their effectiveness across different populations.
RATING
The article provides a timely and relevant discussion on the challenges and potential of AI in healthcare, particularly focusing on the issues of data silos and the need for diverse data training. It presents a balanced view, acknowledging both the transformative potential of AI and the significant hurdles it faces. The article is clear and accessible, making complex topics understandable to a broad audience.
However, the article's impact and credibility could be enhanced by incorporating specific examples, case studies, or references to empirical research. This would provide a stronger foundation for the claims made and offer readers a more comprehensive understanding of the issues discussed. Additionally, greater transparency regarding sources and potential conflicts of interest would further strengthen the article's reliability.
Overall, the article contributes positively to the ongoing dialogue about AI in healthcare and encourages stakeholders to consider the broader implications of AI implementation. By addressing these areas, the article could have a more significant impact and engage a wider audience in meaningful discussions about the future of AI in medicine.
RATING DETAILS
The article presents a well-grounded discussion on the limitations of AI in healthcare, focusing on the issues of data silos and the need for diverse data training. The claim that AI models are only as good as the data they are trained on is widely supported in the field of AI and healthcare. The article accurately identifies the problem of overfitting and the risks associated with training AI models on localized data, which is a well-documented issue in machine learning.
However, while the article makes several valid points, it lacks specific data or studies to back up some of its claims. For instance, the assertion that AI models trained in specific regions may not generalize well to others is plausible but would benefit from empirical evidence or case studies. The mention of federated learning as a solution is accurate, yet the article does not provide examples of its successful implementation in healthcare.
Overall, the article is factually accurate in its broad claims, but it would be strengthened by more detailed evidence and references to specific studies or examples. The need for verification of the prevalence of data silos and the effectiveness of federated learning are areas where the article could improve its accuracy.
The article provides a balanced view of the potential and challenges of AI in healthcare. It acknowledges the transformative potential of AI while also critically examining the limitations imposed by data silos. This dual perspective helps to present a comprehensive view of the current state of AI in healthcare.
However, the article primarily focuses on the challenges and risks associated with AI, such as overfitting and bias, without equally highlighting the successful implementations or advancements in AI healthcare applications. This could lead to a slightly skewed perception that the challenges outweigh the benefits, which may not be the case in all scenarios.
The piece could improve its balance by including more examples of where AI has successfully overcome these challenges, or by discussing ongoing efforts and research aimed at mitigating these issues. This would provide a more rounded perspective on the topic.
The article is generally clear and well-structured, making it accessible to a broad audience. It effectively communicates complex issues related to AI in healthcare, such as data silos, overfitting, and bias, in a manner that is easy to understand.
The language used is straightforward and avoids excessive jargon, which helps in maintaining the reader’s engagement and comprehension. The article follows a logical flow, starting with the potential of AI in healthcare, followed by the challenges it faces, and concluding with possible solutions.
However, the article could improve clarity by providing examples or case studies to illustrate its points. This would help readers better grasp the real-world implications of the issues discussed and enhance the overall clarity of the piece.
The article does not cite specific sources or studies, which affects its source quality score. While the author, Sanjay Juneja, M.D., is presented as a credible figure due to his professional background, the lack of external references makes it difficult to assess the reliability of the claims made.
The article would benefit from referencing studies, expert opinions, or data from reputable organizations to support its claims. This would enhance the credibility of the information presented and provide readers with a clearer understanding of the evidence backing the article's assertions.
Incorporating a variety of sources, such as academic research, industry reports, or expert interviews, would significantly improve the source quality and provide a more robust foundation for the article's claims.
The article is transparent in its discussion of the limitations and challenges of AI in healthcare, clearly outlining the issues of data silos and the need for diverse data training. The author’s professional background is mentioned, which provides some context for his perspective.
However, the article lacks transparency in terms of the methodology or evidence supporting its claims. Without citations or references to specific studies, it is difficult for readers to assess the basis of the claims made. Additionally, the article does not disclose any potential conflicts of interest, such as the author's affiliations with AI companies or healthcare organizations, which could impact the impartiality of the analysis.
Improving transparency by providing citations and disclosing any relevant affiliations or potential conflicts of interest would enhance the article's credibility and allow readers to better evaluate the information presented.
Sources
- https://www.healthcatalystcapital.com/documents/FG/healthcatalyst/press/633491_HCC_Life_Sciences_Data_Research_05.23.24.pdf
- https://cyferd.com/patient-safety-threat-the-consequences-of-data-silos-in-healthcare/
- https://pmsquare.com/resource/blogs/2024-6-24-breaking-down-data-silos-and-their-impact-on-ai/
- https://www.healthcatalyst.com/learn/insights/improving-healthcare-data-security-with-ai
- https://www.weforum.org/stories/2025/01/the-energy-paradox-in-healthcare-how-to-balance-innovation-with-sustainability/
YOU MAY BE INTERESTED IN
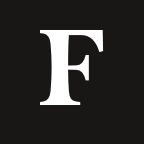
16 Ways Mobile Devices Can Support Health And Wellness
Score 6.2
This AI is creating cancer-killing molecules
Score 6.8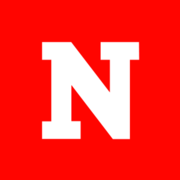
UnitedHealthcare 'Pushing' Boundaries of Medicare Fraud, Republican Says
Score 7.2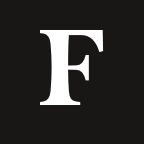
The Commodification Of AI: How Reduced LLM Costs Are Reshaping Healthcare And Beyond
Score 6.0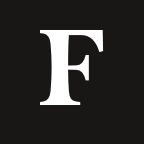