Predictive AI Too Hard To Use? GenAI Makes It Easy

Generative AI (GenAI) is transforming the accessibility of predictive AI by offering an intuitive interface for business professionals, allowing them to leverage the power of predictive models without needing extensive technical knowledge. By employing AI chatbots configured as virtual data scientists, businesses can tackle predictive AI projects more effectively. These chatbots simplify complex concepts, help interpret profit curves, and offer strategic insights, enabling users to make informed decisions on marketing campaigns and other operational tasks. This approach addresses a significant barrier in the deployment of predictive AI, which often requires data scientists and technical know-how.
The integration of GenAI into predictive AI projects exemplifies the potential synergy between these two AI fields, promising broader accessibility and application. This advancement not only assists in overcoming the technical challenges associated with predictive AI but also helps bridge the gap between technical experts and business users. By improving understanding and accessibility, GenAI can enhance the effectiveness of predictive AI, leading to more successful project deployments and unlocking untapped potential in business operations. This development could redefine how businesses utilize AI, expanding its impact across various sectors.
RATING
The article provides a clear and engaging overview of the potential for generative AI to enhance the accessibility and effectiveness of predictive AI in business contexts. It excels in clarity and readability, using relatable examples to explain complex concepts. However, the article could benefit from greater transparency, source quality, and balance by including more diverse perspectives and empirical evidence to support its claims. While the topic is timely and relevant, addressing potential controversies and ethical considerations could enhance its depth and impact. Overall, the article serves as a thought-provoking introduction to the integration of different AI technologies, though it would be strengthened by a more comprehensive exploration of the challenges and limitations involved.
RATING DETAILS
The article accurately highlights the complexity of predictive AI and its general inaccessibility to non-technical business users. It correctly notes that predictive AI often requires the expertise of data scientists to be effectively utilized. However, the claim that generative AI (genAI) can make predictive AI more accessible is more speculative and would benefit from empirical evidence or case studies demonstrating this capability. The example of using a genAI chatbot to explain a marketing profit curve is plausible, but it is presented without direct evidence or detailed case study validation. The article also suggests that predictive AI offers more value than generative AI, a statement that is context-dependent and requires more nuanced discussion across different industries and applications.
The article primarily presents a positive view of how generative AI can assist predictive AI, potentially overlooking challenges or limitations in this integration. It lacks diverse perspectives, such as those from critics who might question the feasibility or effectiveness of using genAI in this manner. By focusing predominantly on the benefits, the article may unintentionally underrepresent the technical challenges or the learning curve associated with implementing such AI solutions in real-world business scenarios. The article could be more balanced by including insights from industry experts or case studies that highlight both successes and failures in this area.
The article is generally clear and well-structured, with a logical flow that guides the reader through the main points. The use of a relatable example, such as the lemonade stand analogy, helps demystify complex concepts and makes the content accessible to a broader audience. However, while the language is straightforward, some technical terms and concepts might still be challenging for readers without a background in AI. Overall, the article does a good job of explaining the potential role of genAI in predictive AI projects, though it could benefit from further simplification or definitions of technical jargon.
The article does not cite specific sources or experts, which limits the assessment of its source quality. It mentions Dean Abbott as a contributor to the example used in the article, but it does not provide sufficient context or references to validate the claims made. The lack of attributed sources makes it difficult to evaluate the authority and reliability of the information presented. To enhance source quality, the article could include references to studies, expert opinions, or data that support its claims about the accessibility and value of predictive AI.
The article lacks transparency in terms of its methodology and the basis for its claims. It does not disclose the background or qualifications of the author, nor does it explain the process by which the conclusions were reached. There is no mention of potential conflicts of interest or biases that might affect the article's impartiality. Greater transparency could be achieved by providing more context about the sources of information, the author's expertise, and any affiliations that could influence the article's perspective.
Sources
- https://binmile.com/blog/generative-ai-vs-predictive-ai/
- https://www.eweek.com/artificial-intelligence/generative-ai-vs-predictive-ai/
- https://lumenalta.com/insights/10-key-differences-between-generative-ai-and-predictive-ai
- https://www.ibm.com/think/topics/generative-ai-vs-predictive-ai-whats-the-difference
- https://www.techtarget.com/searchenterpriseai/tip/Generative-AI-vs-predictive-AI-Understanding-the-differences
YOU MAY BE INTERESTED IN
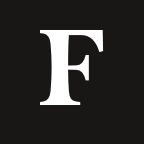
Agentic, Generative And Predictive: How The AI Orchestra Works In Harmony
Score 6.8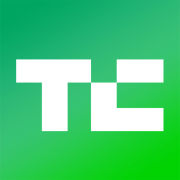
Character.AI unveils AvatarFX, an AI video model to create lifelike chatbots
Score 6.0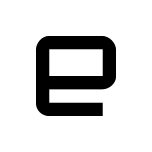
Using generative AI will 'neither help nor harm the chances of achieving' Oscar nominations
Score 6.8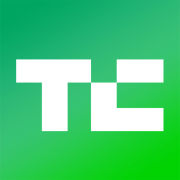
Canva is getting AI image generation, interactive coding, spreadsheets and more
Score 7.2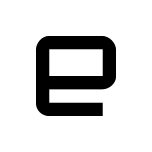