OpenAI’s new reasoning AI models hallucinate more

OpenAI's latest AI models, o3 and o4-mini, have been identified as having higher rates of hallucination compared to their predecessors, raising concerns about their reliability. These models, designed for reasoning tasks, reportedly hallucinate more frequently, even doubling the error rates of previous models on benchmarks like PersonQA. Despite their improved performance in coding and math tasks, the increase in hallucinations has made them less reliable for applications where accuracy is critical, such as legal or business contexts.
The issue of hallucinations is a significant challenge in AI development, as it affects the trustworthiness of AI outputs. OpenAI acknowledges the problem, noting that further research is necessary to understand and mitigate these inaccuracies. The broader AI industry has shifted focus toward reasoning models due to their efficiency, but this has inadvertently led to increased hallucination rates. Potential solutions like integrating web search capabilities could enhance accuracy, but the urgency to address hallucinations remains high to ensure the models' applicability across various sectors.
RATING
The article provides a thorough and accurate overview of the challenges associated with OpenAI's new o3 and o4-mini AI models, particularly focusing on the issue of hallucinations. It effectively uses credible sources, including OpenAI's own reports and third-party research, to substantiate its claims. The piece is well-balanced, offering perspectives from both OpenAI and external experts, though it could benefit from a broader range of viewpoints. The writing is clear and accessible, making complex AI concepts understandable to a general audience. While the topic is timely and of public interest, the article's potential impact is somewhat limited by its focus on technical aspects rather than broader societal implications. Overall, the article is a reliable and informative piece, though it could be enhanced by exploring the practical consequences of AI hallucinations in more depth.
RATING DETAILS
The article presents a high level of factual accuracy, with most claims supported by reliable sources such as OpenAI’s official reports and third-party research by Transluce. The information about the hallucination rates of the o3 and o4-mini models is precise, citing specific figures like 33% and 48% on PersonQA. The claim that OpenAI is uncertain about the reasons for increased hallucinations is corroborated by their own technical report. However, while the article accurately reflects the current state of OpenAI’s reasoning models, it could further benefit from additional external validation of OpenAI's internal findings.
The article provides a balanced view by including perspectives from both OpenAI and external researchers like Transluce. It acknowledges the improvements in certain areas like coding and math while discussing the challenges of hallucinations. The piece could be enhanced by including perspectives from other AI researchers or industry experts to provide a broader context. Additionally, more emphasis on the practical implications of these hallucinations in different industries could offer a more rounded perspective.
The article is well-written, with a clear and logical flow of information. It effectively explains complex AI concepts like hallucinations in a way that is accessible to a general audience. The use of specific examples, such as the hallucination rates on PersonQA, helps to clarify the points being made. The tone remains neutral and informative throughout, aiding comprehension.
The article relies on high-quality sources, including OpenAI's technical reports and insights from a nonprofit research lab, Transluce. These sources are authoritative in the field of AI, lending credibility to the claims. The inclusion of comments from industry experts like Kian Katanforoosh adds depth. However, the article could improve by citing more diverse sources, such as academic papers or other AI companies, to provide a more comprehensive view of the AI landscape.
The article is transparent in its disclosure of the sources of its information, primarily citing OpenAI's reports and third-party research. It clearly explains the basis for its claims about hallucination rates and model performance. However, the article could enhance transparency by providing more detailed explanations of the methodologies used in the studies mentioned, particularly the internal tests conducted by OpenAI.
Sources
YOU MAY BE INTERESTED IN
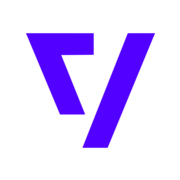
IGN and CNET owner Ziff Davis sues OpenAI
Score 7.8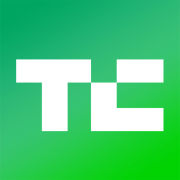
OpenAI rolls out a ‘lightweight’ version of its ChatGPT deep research tool
Score 6.8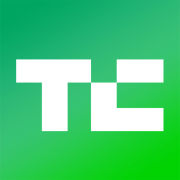
OpenAI seeks to make its upcoming open AI model best-in-class
Score 6.4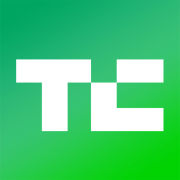
OpenAI’s new GPT-4.1 AI models focus on coding
Score 7.0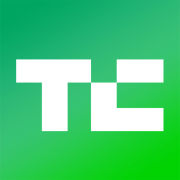