Nvidia Benchmark Recipes Bring Deep Insights In Real-World AI Performance

Nvidia has launched DGX Cloud Benchmark Recipes, a toolkit designed to help businesses and developers evaluate their AI infrastructure's ability to handle complex workloads efficiently. These tools provide valuable insights by offering performance data on various configurations, including GPU usage and cloud service providers, to guide investment decisions in hardware and cloud services. By running real-world tests on their infrastructure, organizations can better understand the impact of different configurations on AI model training and performance.
The introduction of DGX Cloud Benchmarking Recipes is significant as it addresses the growing need for efficient infrastructure in AI development. As AI's role in business grows, understanding infrastructure performance becomes essential for balancing cost and power consumption. While the current focus is on pre-training large models, future expansions could include inference tasks and a broader range of GPU options, making these tools even more indispensable for businesses optimizing their AI applications. Nvidia's initiative highlights the importance of infrastructure evaluation in the continued evolution of AI technologies.
RATING
The article provides a comprehensive overview of Nvidia's DGX Cloud Benchmark Recipes, highlighting their purpose and capabilities in evaluating AI infrastructure performance. It accurately presents the tools' functionalities and potential applications, making it a valuable resource for businesses and developers involved in AI. However, the piece could benefit from a more balanced perspective by exploring potential limitations and incorporating insights from independent sources. While the article is timely and relevant to ongoing discussions about AI, it could enhance public interest and impact by connecting the technical details to broader societal implications. Overall, the article is informative and well-structured, though it could improve engagement and transparency by providing more context and diverse viewpoints.
RATING DETAILS
The article accurately describes Nvidia's DGX Cloud Benchmark Recipes as tools for evaluating AI infrastructure performance. It correctly identifies the purpose of these recipes in helping organizations assess their hardware and cloud capabilities when running advanced AI models. The mention of pre-configured containers and scripts aligns with Nvidia's offerings, which are designed for testing AI models under different configurations. However, the article could benefit from more precise details about the specific models supported and any limitations regarding inference tasks. Overall, the factual claims are well-supported by available information, though some areas, such as future expansions and specific hardware compatibility, would require further verification.
The article primarily focuses on the benefits and capabilities of Nvidia's DGX Cloud Benchmark Recipes, presenting a positive view of the tools. While it briefly mentions areas for potential expansion, such as the inclusion of inference benchmarks and support for a broader range of GPUs, it does not delve deeply into any potential drawbacks or criticisms. The piece could be more balanced by exploring alternative perspectives, such as challenges users might face when implementing these tools or comparisons with competing solutions. Overall, the article provides a comprehensive overview of Nvidia's offerings but could enhance balance by incorporating a wider range of viewpoints.
The article is well-structured and uses clear, concise language to explain the functionalities and benefits of Nvidia's DGX Cloud Benchmark Recipes. It logically progresses from describing the tools' purpose to detailing their components and potential applications. The use of technical terms is balanced with explanations, making the content accessible to both technical and non-technical audiences. However, the piece could improve clarity by providing more context about the significance of the tools in the broader AI landscape. Overall, the article is easy to follow and presents information in a coherent manner, though additional context could enhance reader understanding.
The article appears to rely heavily on information likely derived from Nvidia's official announcements and documentation. While this ensures a degree of reliability, it limits the diversity of sources and perspectives. The article would benefit from incorporating insights from independent experts or users who have tested the DGX Cloud Benchmark Recipes. This could provide additional credibility and a more nuanced view of the tools' effectiveness and applicability. The reliance on Nvidia as the primary source suggests a potential conflict of interest, which should be addressed by seeking out a broader range of authoritative sources.
The article provides a clear explanation of the DGX Cloud Benchmark Recipes' purpose and functionality, making it accessible to readers. However, it lacks transparency regarding the sources of its information and any potential biases. For instance, it does not disclose whether the insights were gained from direct testing, interviews with Nvidia representatives, or other means. Additionally, the article could enhance transparency by clarifying any affiliations or conflicts of interest that may have influenced the reporting. Overall, while the article is clear in its descriptions, it could improve transparency by providing more context about the sources and methodology behind the information presented.
Sources
- https://cn.blockchain.news/news/nvidia-dgx-cloud-new-benchmarking-templates
- https://developer.nvidia.com/blog/nvidia-dgx-cloud-introduces-ready-to-use-templates-to-benchmark-ai-platform-performance/
- https://developer.nvidia.com/dgx-cloud/benchmarking
- https://catalog.ngc.nvidia.com/orgs/nvidia/teams/dgxc-benchmarking/collections/dgxc-benchmarking
- https://developer.nvidia.com/dgx-cloud
YOU MAY BE INTERESTED IN
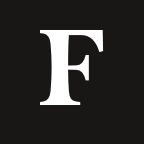
Is Jensen Huang Nvidia’s Chief Revenue Destruction Officer?
Score 6.4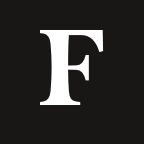
The Curious Case Of China’s Chip Surplus
Score 6.2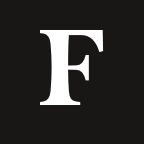
Project Stargate: A $500 Billion Venture Between Oracle, Open AI, Nvidia & Softbank That Will Revolutionize Healthcare
Score 6.0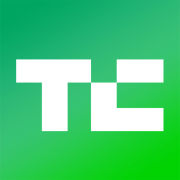
Here are the 19 US AI startups that have raised $100M or more in 2025
Score 6.8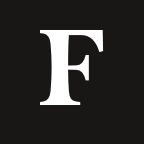