Data Quality As The Missing Piece In AI And Space Strategies

In the rapidly evolving field of artificial intelligence, the need for high-quality earth observation (EO) data is becoming increasingly critical. Elizabeth Duffy, Product Marketing Director at EarthDaily, highlights the importance of reliable EO data for enhancing AI and machine learning applications across various sectors. The proliferation of low Earth orbit satellites has increased EO data availability, yet challenges such as data quality inconsistencies, limited spectral diversity, and sensor noise persist. Improving data quality can lead to more accurate predictions, better model explainability, and significant impacts in agriculture, disaster response, and financial services.
The implications of investing in high-quality EO data are profound, as it enhances AI model performance and reduces costs. Organizations prioritizing data quality can improve decision-making and market positioning. With the global data volume expected to nearly double by 2026, robust AI techniques are essential for optimal resource use. As EO data becomes a vital component of modern AI systems, businesses that embrace this technological synergy will unlock new opportunities for innovation and growth.
RATING
The article presents an important discussion on the role of data quality in AI systems, particularly through the lens of Earth Observation data. Its strengths lie in highlighting the relevance of high-quality data for AI applications across various sectors, which is a timely and pertinent topic. However, the article's effectiveness is somewhat diminished by a lack of source attribution, limited transparency, and an absence of balanced perspectives. While it successfully communicates the potential benefits of integrating EO data with AI, it could be strengthened by providing more robust evidence, diverse viewpoints, and clearer explanations of technical concepts. Overall, the article serves as an informative introduction to the topic but would benefit from a more comprehensive approach to enhance its credibility and impact.
RATING DETAILS
The article makes several factual claims about the importance and impact of high-quality data in AI systems, particularly Earth Observation (EO) data. While the general principles discussed, such as the necessity of quality data for AI model performance, are widely accepted, specific figures and claims require verification. For instance, the claim that the combined revenue of AI use cases for image recognition, algorithmic trading strategies, and localization and mapping is $20 billion needs support from market research or financial reports. Additionally, the assertion that $3.1 trillion of America's GDP is lost due to poor-quality data should be backed by official IBM sources or related studies. The article's accuracy is somewhat compromised by the lack of direct citations or references to authoritative sources for these claims.
The article primarily presents a positive view of the integration of EO data with AI, emphasizing the benefits and potential applications. However, it lacks a balanced perspective by not sufficiently addressing the challenges or limitations associated with this integration. For example, while it mentions data quality issues like sensor noise and limited spectral diversity, it does not explore the potential downsides or risks of relying heavily on EO data. The article could have been more balanced by including perspectives from experts who may have reservations or concerns about the current state of EO data quality and its implications for AI.
The article is generally clear in its language and structure, with a logical flow that guides the reader through the importance of data quality in AI systems. The tone is neutral and informative, making it accessible to a general audience. However, some technical terms and concepts, such as spectral reflectance and signal-to-noise ratio, might require further explanation for readers unfamiliar with geospatial analysis. Overall, the article successfully communicates its main points, but could benefit from additional clarification of complex terms.
The article does not provide clear attributions to specific sources for many of its claims, which affects the perceived credibility and reliability of the information presented. While it references a Gartner report and an IBM claim, it does not provide direct links or citations to these sources. Additionally, the article lacks a variety of sources, relying heavily on generalized statements without supporting evidence from authoritative or diverse sources. This lack of attribution and source variety undermines the article's overall source quality.
The article lacks transparency in terms of disclosing the basis for its claims and the methodology behind the data presented. It does not provide sufficient context or explanations for how certain figures, such as the $20 billion revenue from AI use cases, were derived. Additionally, there is no disclosure of potential conflicts of interest that could impact the impartiality of the information, such as the author's affiliation with EarthDaily. Greater transparency in these areas would enhance the article's credibility and trustworthiness.
YOU MAY BE INTERESTED IN
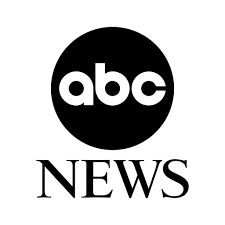
Lawsuit against Florida ban on 'lab-grown' meat still alive after judge's ruling
Score 6.8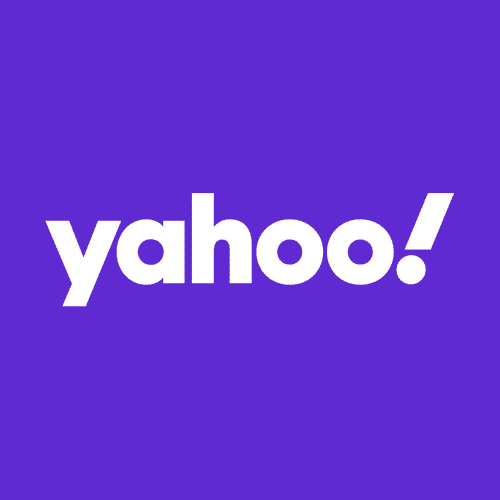
North Dakota governor signs bill providing legal protections for pesticides manufacturers
Score 8.0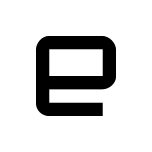
Strava can predict your race finish times
Score 7.0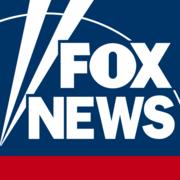
Robotic and drone tech make fruit picking and handling easier
Score 6.4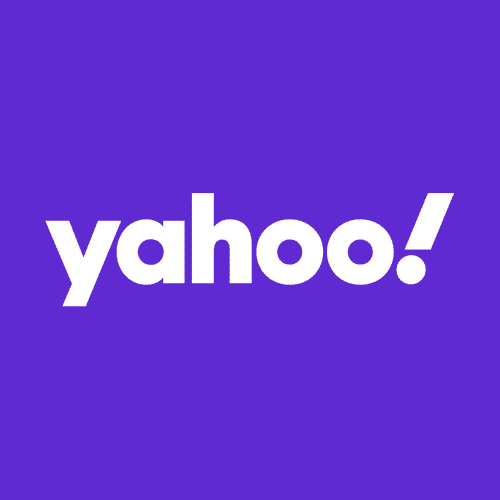