Data Engineering: Transforming The Backbone Of Modern Data Solutions

Mukul Garg, Head of Support Engineering at PubNub, highlights a pivotal shift in data engineering as companies integrate real-time data pipelines to enhance business agility. By adopting technologies like Apache Kafka and cloud-native solutions, organizations such as Netflix, Uber, Shopify, and Airbnb have transformed their data processing capabilities. This shift allows for immediate data insights, improved decision-making, and personalized customer experiences, underscoring the critical role of data engineering in digital transformation strategies.
The story contextualizes the significance of modern data engineering in today's data-driven world. As the complexity of data sources grows, the demand for real-time analytics propels businesses to implement scalable and efficient data infrastructures. While the benefits are substantial—real-time analytics, scalability, data democratization, and cost efficiency—challenges such as data quality, privacy compliance, and integration complexity remain. Looking forward, trends like data fabric expansion, serverless platforms, and privacy by design will further shape the future of data engineering, positioning companies to gain a competitive edge.
RATING
The article provides a comprehensive overview of the role of data engineering in modern digital solutions, highlighting its benefits and challenges. It effectively uses examples from well-known companies to illustrate its points, making the narrative engaging and relatable for industry professionals. However, the lack of direct citations and transparency in sourcing diminishes the overall credibility and reliability of the information presented.
While the article is timely and relevant to ongoing technological advancements, its focus on positive outcomes and successful implementations creates an imbalance, as it does not equally address the potential pitfalls or failures that can occur. The readability and clarity of the article are strong, making it accessible to those with a basic understanding of technology, but it could benefit from additional explanations of technical terms for a broader audience.
Overall, the story is informative and provides valuable insights into the future of data engineering, but it would be strengthened by greater transparency, a more balanced perspective, and more robust sourcing.
RATING DETAILS
The story provides a detailed account of the role of data engineering in modern digital solutions, citing examples from major companies like Netflix, Uber, Shopify, and Airbnb. The factual accuracy of these claims largely hinges on the verifiability of the technologies and methodologies mentioned, such as Netflix's combination of batch and stream processing or Uber's predictive analytics engine. These claims align well with known industry practices, but specific details about implementation and impact are not thoroughly verified within the text.
For instance, the claim that Shopify moved to an automated data warehouse using cloud-native solutions like dbt is plausible and aligns with industry trends, but the article does not provide direct evidence or citations to confirm this. Similarly, assertions about Airbnb's adoption of a data mesh architecture are consistent with current technological advancements but lack direct verification. The story's accuracy could be enhanced with more precise data points or references to external sources that substantiate these claims.
The story predominantly highlights the positive impacts of modern data engineering, focusing on efficiency, scalability, and real-time analytics. While it acknowledges challenges such as data quality and integration complexity, these are somewhat overshadowed by the success stories of large tech companies. This creates a potential imbalance, as the narrative leans heavily towards the benefits without equally addressing the difficulties or failures some companies might face.
Moreover, the perspectives included are mainly from successful implementations in large, well-known companies. There is a lack of viewpoints from smaller businesses or those that have struggled with similar technological transformations. Including a broader range of experiences could provide a more balanced view of the data engineering landscape.
The article is well-structured and uses clear language to convey complex technical concepts, making it accessible to a broad audience. The logical flow from the introduction of data engineering concepts to specific company examples helps in understanding the narrative. The use of subheadings and bullet points further aids in the readability and comprehension of the content.
However, while the article is clear in describing the benefits of data engineering, it could provide more context or definitions for some of the more technical terms used, such as 'data mesh architecture' or 'serverless data platforms.' This would ensure that readers without a technical background can fully grasp the concepts discussed.
The article does not explicitly cite sources or provide direct quotes from experts, which makes it difficult to assess the credibility and reliability of the information presented. The lack of attribution to specific studies, reports, or expert opinions reduces the authority of the claims made, particularly those regarding industry trends and technological implementations.
While the companies mentioned are reputable and known for their technological advancements, the absence of direct references or links to company reports or external analyses diminishes the overall source quality. Including a variety of authoritative sources would enhance the article's credibility and provide a more robust foundation for the claims.
The article lacks transparency in terms of the methodology used to gather information and the basis for the claims made. There is no disclosure of how the information was obtained, whether through interviews, company reports, or independent research. This lack of transparency makes it challenging for readers to assess the impartiality and reliability of the content.
Additionally, there is no mention of potential conflicts of interest or biases that might influence the narrative. For example, the author’s connection to PubNub, a company involved in data solutions, is stated but not explored in terms of how it might affect the article's perspective. Greater transparency about the sources of information and any potential biases would improve the article's trustworthiness.
Sources
- https://www.confluent.io/learn/streaming-data-pipelines/
- https://www.tinybird.co/blog-posts/real-time-data-platforms
- https://www.snowflake.com/en/solutions/use-cases/snowflake-streaming-data-pipelines/
- https://architecture.arcgis.com/en/framework/system-patterns/real-time-data-streaming-and-analytics/deployment-patterns/as-saas.html
- https://estuary.dev/product/
YOU MAY BE INTERESTED IN
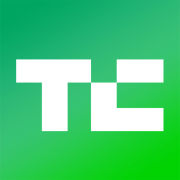
DoorDash seeks dismissal of Uber lawsuit
Score 7.2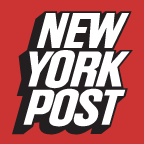
7 Shows Like ‘Ransom Canyon’ If You’re Craving More Soapy Sagas Or Small-Town Streams
Score 6.0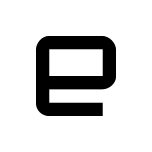
Netflix subtitles are now available in a dialogue-only format
Score 7.8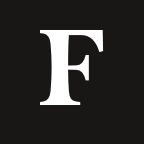
If OpenAI Buys Chrome, AI May Rule The Browser Wars
Score 7.2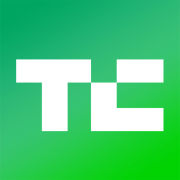