Building Enterprise AI On A Granite Foundation Of Trust And Confidence

Enterprises implementing AI systems must prioritize security, privacy, and compliance alongside cost and performance. Recent developments highlight the need for robust AI strategies, as safety concerns around models like DeepSeek R1, which is prone to jailbreak attacks and biases, emerge. IBM’s Granite 3.2 models, however, claim to maintain security and trust while offering cost-effective solutions, showcasing the balance needed between performance and integrity.
The implications of these findings are significant, as businesses must conduct comprehensive risk assessments when selecting AI models. While DeepSeek R1 offers economic advantages, its vulnerabilities underscore the necessity of thorough security evaluations. IBM’s approach with Granite 3.2, including the use of guardrail models like Granite Guardian, emphasizes the importance of safety and compliance in AI applications. The overall takeaway for enterprises is to evaluate AI models not just on cost per token but on the total cost of ensuring operational integrity and security.
RATING
The article provides a timely and relevant discussion of enterprise AI, focusing on the importance of trust, security, and compliance. It effectively highlights the challenges and considerations enterprises face when selecting AI models, using specific examples to illustrate its points. However, the article's accuracy and credibility are somewhat limited by the lack of direct citations and independent sources, which affects the strength of its claims.
While the article is generally clear and well-structured, it could benefit from greater transparency in its sources and methodologies, as well as a more balanced representation of perspectives. By incorporating a wider range of viewpoints and providing more robust evidence, the article could enhance its impact and engagement potential.
Overall, the article contributes to ongoing discussions about AI ethics and security but could improve its quality by addressing the limitations in its source quality and balance.
RATING DETAILS
The article presents several claims that are factually complex, requiring verification to ensure accuracy. For instance, it claims that DeepSeek R1 is highly vulnerable to jailbreak attacks, citing a study by Cisco, but does not provide direct evidence or a source for this assertion, making it difficult to verify. Additionally, the claim of a 'strong China bias' in DeepSeek R1 lacks detailed evidence or context, which raises questions about its precision and truthfulness.
Another key claim is that IBM's Granite 3.2 models maintain safety and robustness while delivering reasoning performance. While the article cites IBM's own assertions and the AttaQ benchmark results, independent verification of these claims would strengthen their credibility. The cost comparison between DeepSeek R1 and IBM's models is another area that requires precise data and context to ensure the figures are accurate and not misleading.
Overall, while the article provides a detailed discussion of AI model security and performance, the lack of direct sources for some claims and the need for external verification of others somewhat limit its factual accuracy.
The article predominantly discusses the advantages of IBM's Granite 3.2 models over DeepSeek R1, which may indicate a potential imbalance in perspective. While it acknowledges the cost-efficiency of DeepSeek R1, it focuses heavily on its vulnerabilities and biases, potentially downplaying any positive aspects or improvements made by DeepSeek.
Moreover, the article does not seem to explore other AI models outside of DeepSeek and IBM, which could offer a more comprehensive view of the enterprise AI landscape. The lack of diverse viewpoints or counterarguments to the claims made about IBM's models contributes to a somewhat one-sided narrative.
By not including perspectives from independent experts or users of these AI models, the article may miss important insights into the practical applications and limitations of these technologies.
The article is generally clear in its language and structure, making it accessible to readers with a basic understanding of AI technologies. It logically outlines the considerations for enterprise AI, such as cost, performance, and security, and provides specific examples of AI models to illustrate these points.
However, the article could benefit from clearer explanations of technical terms and concepts, such as 'jailbreak attacks' or 'chain-of-thought reasoning,' to ensure that all readers, regardless of their technical expertise, can fully understand the implications.
Overall, while the article is well-structured, it could improve its clarity by simplifying complex ideas and providing more context for technical terms.
The article lacks direct citations or references to external studies, reports, or expert opinions, which undermines the credibility of its claims. For example, the mention of a Cisco study on DeepSeek R1's vulnerabilities is not accompanied by a link or reference, making it difficult to assess the study's methodology or validity.
Additionally, the article relies heavily on IBM's assertions regarding its Granite models, without incorporating independent evaluations or third-party assessments. This reliance on potentially biased sources can affect the impartiality of the reporting.
A more robust variety of sources, including independent studies or expert analyses, would enhance the reliability and depth of the information presented.
The article provides some context about the importance of trust and security in enterprise AI but lacks transparency in explaining the basis for certain claims. For instance, the article does not disclose the methodology or specific criteria used in the Cisco study or the AttaQ benchmark, which are critical for understanding the validity of the results cited.
Additionally, the article does not address potential conflicts of interest, such as whether the author or publication has any affiliations with the companies mentioned, which could impact the neutrality of the information.
Greater transparency in disclosing sources, methodologies, and potential biases would improve the article's credibility and help readers better assess the information.
Sources
- https://www.modelop.com/blog/trust-is-the-linchpin-to-enterprise-ai-success-and-roi
- https://www.businesswire.com/news/home/20250307228515/en/Fairmarkit-Named-to-Forbes%E2%80%99-2025-List-of-Americas-Best-Startup-Employers
- https://narwalinc.com/the-future-of-enterprise-ai-how-businesses-can-leverage-ai-for-growth/
- https://www.teradata.com/resources/articles/enterprises-need-to-put-ia-before-ai
- https://www.youtube.com/watch?v=q__urR1_yCU
YOU MAY BE INTERESTED IN
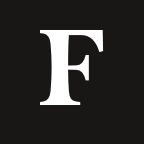
Google Cloud’s Vertex And Models Advance Enterprise AI Agent Adoption
Score 7.2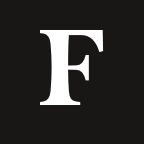
IBM’s Enterprise AI Strategy: Trust, Scale, And Results
Score 6.6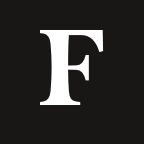
Pure Storage Introduces FlashBlade//EXA For AI And HPC
Score 6.8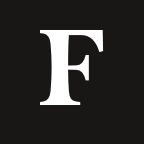
Reinventing AI’s Future: The Ecosystem Dilemma
Score 6.2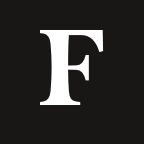