Blockchain-Proven Content And Nvidia Alternative? Check Out CrowdGenAI

CrowdGenAI, led by CEO Dr. Sam Khoze and COO Susan Koehler, is emerging as a formidable competitor to Nvidia by offering a CPU-based AI platform that matches GPU efficiency in AI training while significantly reducing costs and energy consumption. This breakthrough was unveiled at the World Economic Forum 2025 in Davos. CrowdGenAI's innovative approach utilizes existing CPU clusters, making AI accessible and sustainable, all while integrating blockchain-based watermarking for data ownership and provenance. This development is gaining attention from tech giants like Google and Microsoft, who are partnering with CrowdGenAI to drive AI innovation and sustainability.
The rise of AI technologies has exposed challenges in data ownership and environmental sustainability. Nvidia's GPUs, though powerful, are costly and energy-intensive, contributing to a growing carbon footprint. CrowdGenAI addresses these issues by shifting AI workloads onto CPU infrastructure, reducing reliance on power-hungry GPUs and promoting ethical AI governance through its TraceID system. By providing a decentralized, transparent AI ecosystem, CrowdGenAI not only challenges Nvidia's dominance but also offers businesses a path to more environmentally friendly and ethically governed AI solutions.
RATING
The article presents an intriguing narrative about CrowdGenAI's potential to revolutionize AI technology through CPU-based systems and blockchain-based watermarking. While it highlights significant challenges in the AI industry, such as sustainability and data ownership, the article falls short in providing verifiable evidence and diverse perspectives to substantiate its claims. The lack of external sources and detailed data limits the credibility and impact of the story. Despite these shortcomings, the article effectively communicates complex technical concepts in an accessible manner and addresses timely issues that are of public interest. To enhance its reliability and engagement potential, the article would benefit from incorporating third-party validation, detailed case studies, and a more balanced presentation of viewpoints.
RATING DETAILS
The article makes several factual claims about CrowdGenAI, such as its ability to match Nvidia's GPUs in AI training efficiency using optimized CPU clusters and its blockchain-based watermarking system. However, these claims require verification through independent studies or technical documentation. The article lacks specific benchmarks or studies to substantiate the performance comparison between CPU clusters and Nvidia GPUs. Additionally, the claim of reducing energy consumption by up to 50% is not supported by detailed data or case studies. The mention of partnerships with major companies like Google and Microsoft also needs confirmation. Without these verifications, the story's factual accuracy remains uncertain.
The article primarily focuses on the advantages of CrowdGenAI's technology and its potential to disrupt Nvidia's dominance. It does not adequately present counterarguments or potential limitations of CPU-based AI systems. For instance, while it briefly mentions that CPUs may not match the raw speed of GPUs, it does not explore this limitation in depth. The article also lacks perspectives from industry experts or competitors, which could provide a more balanced view of the technology's impact and feasibility.
The article is generally clear in its language and structure, presenting information in a logical sequence. It effectively explains technical concepts, such as blockchain-based watermarking and CPU-based AI training, in an accessible manner. However, some sections could benefit from additional detail to enhance comprehension, particularly regarding the technical aspects of the CPU clusters and their comparison to GPUs. Overall, the article maintains a neutral tone, although it leans towards promoting CrowdGenAI's technology.
The article does not cite any external sources or studies to support its claims, relying heavily on information presumably provided by CrowdGenAI. This lack of source variety and authority raises concerns about the reliability of the information. The absence of third-party validation or expert opinions makes it difficult to assess the credibility of the claims made about the technology's performance and partnerships.
The article lacks transparency in terms of disclosing the basis for its claims. It does not provide details on the methodology used to assess the performance of CPU clusters versus GPUs or the specifics of the blockchain-based watermarking system. Additionally, there is no discussion of potential conflicts of interest, such as whether the author has any affiliations with CrowdGenAI or its partners. This lack of transparency makes it challenging for readers to evaluate the impartiality of the information presented.
Sources
YOU MAY BE INTERESTED IN
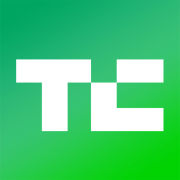
Ironwood is Google’s newest AI accelerator chip
Score 7.0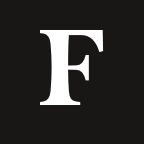
Recent Breakthroughs Accelerate The Race For Quantum Computing
Score 6.6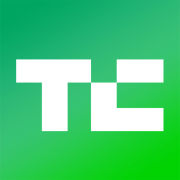
Within six years, building the leading AI data center may cost $200B
Score 5.8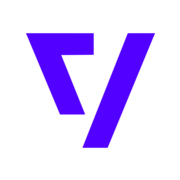
Google is paying Samsung an ‘enormous sum’ to preinstall Gemini
Score 7.2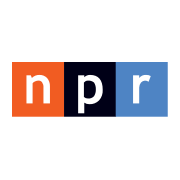