Beyond The Llama Drama: 4 New Benchmarks For Large Language Models

In April 2025, Meta's release of the Llama 4 series of language models sparked a significant controversy in the AI community. The Llama 4 Maverick model was initially celebrated for its top ranking on LMArena, a benchmarking platform. However, it was later revealed that Meta submitted a specially tuned version of Llama 4 Maverick, not identical to the public release, raising accusations of 'benchmark hacking.' Critics and anonymous sources suggested that performance targets were met through potential 'data contamination' by training on data similar to benchmark tests. Meta's VP of Generative AI denied these claims, attributing performance differences to platform-specific tuning. The incident prompted LMArena to revise its evaluation policies, highlighting vulnerabilities in current AI assessment methods.
The Llama 4 controversy underscores broader issues with existing benchmarks for large language models (LLMs). Traditional metrics like MMLU and HumanEval are increasingly seen as insufficient due to data contamination, benchmark overfitting, and a narrow focus on isolated tasks. These limitations fail to capture qualitative aspects like ethical reasoning, empathy, and user interaction quality. As AI models play a more significant role in society, there is a growing call for a more comprehensive evaluation framework that includes human-centric dimensions. This holistic approach aims to ensure AI development aligns with human values and enhances human capability, emphasizing the need for dynamic, adaptive evaluation systems as models evolve.
RATING
The article "Beyond The Llama Drama: 4 New Benchmarks For Large Language Models" provides a comprehensive overview of the controversy surrounding Meta's Llama 4 models and highlights broader issues in evaluating Large Language Models (LLMs). The story is timely, addressing a current and relevant topic in the AI field, and it effectively captures the reader's attention with a compelling narrative.
The article is well-written and easy to understand, with clear language and a logical structure. It presents a balanced view of the Llama 4 controversy, highlighting both the strengths and criticisms of the models. However, the story could improve by incorporating more diverse viewpoints and providing more detailed evidence or examples to support its claims.
Overall, the article successfully addresses a topic of significant public interest, with implications for both AI researchers and the general public. It has the potential to influence public opinion and drive discussions about AI evaluation, but it could enhance its impact by providing more concrete evidence and examples.
RATING DETAILS
The story's accuracy is generally strong, with well-documented claims about the release and controversy surrounding Meta's Llama 4 models. The article accurately describes the allegations of benchmark hacking and data contamination, which are supported by reports from credible sources like ZDNet and The Register. However, the story could improve by providing more specific evidence or examples to support these claims, such as direct quotes from Meta insiders or detailed comparisons of the submitted and public models.
The article's discussion of benchmarking limitations is well-founded, citing common issues like data contamination and overfitting. These claims align with known challenges in evaluating LLMs, but the story could benefit from more precise data or studies to back up these assertions. Additionally, the article proposes new benchmarks for LLMs, which, while innovative, lack specific examples or evidence of their effectiveness.
Overall, the story provides a mostly accurate depiction of the Llama 4 controversy and broader issues in LLM evaluation. However, it could improve by offering more concrete evidence and examples to support its claims.
The story presents a balanced view of the controversy surrounding Meta's Llama 4, highlighting both the impressive capabilities of the models and the criticisms they have faced. It acknowledges the strengths of the Llama 4 suite while also addressing the issues of benchmark hacking and data contamination. However, the article could benefit from incorporating more perspectives, such as Meta's response to the allegations or opinions from independent AI experts.
The article primarily focuses on the limitations of current benchmarks and the need for new evaluation methods, which may give the impression of bias against existing practices. Including a broader range of viewpoints, such as those from researchers who support current benchmarks or alternative evaluation methods, would enhance the story's balance.
Overall, the story provides a reasonably balanced perspective on the Llama 4 controversy but could improve by incorporating more diverse viewpoints and addressing potential biases in its discussion of benchmarking.
The article is well-written and easy to follow, with a logical structure that guides the reader through the Llama 4 controversy and the broader issues in LLM evaluation. The language is clear and accessible, making it suitable for a general audience interested in AI developments.
The story effectively explains complex concepts, such as benchmark hacking and data contamination, in a way that is understandable to readers without a technical background. However, the article could improve clarity by providing more concrete examples or evidence to support its claims, which would help readers better understand the issues discussed.
Overall, the article is clear and well-structured, but it could enhance clarity by providing more specific examples and evidence.
The article cites credible sources like ZDNet and The Register, which are well-regarded in the technology and AI sectors. These sources lend credibility to the claims about the Llama 4 controversy and the broader issues in LLM evaluation. However, the article could improve by providing more direct quotes or evidence from these sources to strengthen its arguments.
The story also references anonymous online posts allegedly from Meta insiders, which could be less reliable. While these claims are plausible, they would benefit from corroboration by named sources or additional evidence. Including expert opinions or studies on the limitations of current benchmarks would also enhance the story's credibility.
Overall, the article's source quality is solid, but it could be strengthened by providing more direct evidence and including a wider range of sources.
The article provides a clear overview of the Llama 4 controversy and the limitations of current benchmarks, but it lacks transparency in certain areas. For instance, it does not specify the sources of some claims, such as the anonymous posts from Meta insiders, which could affect the story's credibility.
The article could improve transparency by clearly outlining the methodology used to evaluate the claims made and by providing more detailed evidence or examples. Additionally, disclosing any potential conflicts of interest or biases in the reporting would enhance the story's transparency.
Overall, the article is reasonably transparent in its presentation of the Llama 4 controversy, but it could improve by providing more detailed evidence and disclosing any potential biases or conflicts of interest.
Sources
- https://ai.meta.com/blog/llama-4-multimodal-intelligence/
- https://blog.getbind.co/2025/04/06/llama-4-comparison-with-claude-3-7-sonnet-gpt-4-5-and-gemini-2-5/
- https://mlcommons.org/2025/04/llm-inference-v5/
- https://www.itpro.com/technology/artificial-intelligence/meta-llama-4-model-launch-benchmarks
- https://techcrunch.com/2025/04/11/metas-vanilla-maverick-ai-model-ranks-below-rivals-on-a-popular-chat-benchmark/
YOU MAY BE INTERESTED IN
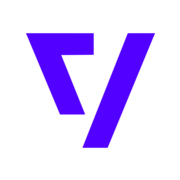
Meta got caught gaming AI benchmarks
Score 6.8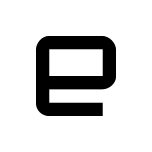
How to watch LlamaCon 2025, Meta's first generative AI developer conference
Score 7.8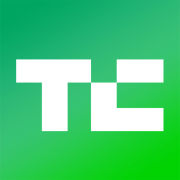
Meta’s vanilla Maverick AI model ranks below rivals on a popular chat benchmark
Score 7.0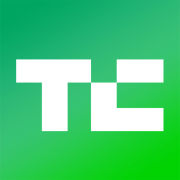
Meta releases Llama 4, a new crop of flagship AI models
Score 6.4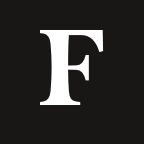