Beyond The Algorithm: Why Data Governance Is Key To Pharma's AI Future

The pharmaceutical industry is on the brink of an AI-driven transformation, with projections indicating that AI-powered drug discovery will become a $9.1 billion market by 2030, growing at a 29.7% CAGR. Key players like Amgen, Bristol Myers Squibb, and Sanofi are already leveraging AI to enhance clinical trials, drug development, and patient recruitment. However, a significant challenge remains: the lack of AI-ready data. The disconnect between AI potential and reality is largely due to issues in data quality, readiness, and technical maturity, with Gartner estimating that 85% of AI projects fail.
To bridge this gap, robust data governance is essential. This involves implementing policies, procedures, and standards to manage data effectively and ethically. Companies must focus on creating a comprehensive data ecosystem architecture, automating data pipelines, upskilling the workforce, and incorporating data privacy and regulatory requirements. By prioritizing these areas, the pharma industry can harness AI's full potential to accelerate drug discovery, personalize treatments, and improve patient outcomes. With AI, what once took months can now be achieved in minutes, signaling a data-driven future for pharmaceuticals.
RATING
The article provides an insightful overview of the potential of AI in the pharmaceutical industry, emphasizing its transformative capabilities in drug discovery and patient treatment. It effectively highlights the importance of data governance and the challenges of implementing AI technologies. However, the article's strength is somewhat undermined by the lack of direct citations and detailed exploration of ethical concerns. While it presents a clear and timely discussion, the absence of diverse perspectives and in-depth analysis of potential risks limits its balance and impact. Overall, the article is a valuable starting point for understanding AI's role in healthcare but would benefit from greater transparency and engagement with critical debates.
RATING DETAILS
The story presents several factual claims, such as the projection that AI-powered drug discovery will become a $9.1 billion market by 2030 and that AI could generate $350 billion to $410 billion in annual value for the pharmaceutical sector by 2025. These claims are significant and require verification from credible sources to ensure accuracy. The article also mentions specific achievements by companies like Amgen and Bristol Myers Squibb in using AI, which could be verified through company reports or press releases. However, the claim that 85% of AI projects fail, attributed to Gartner, is a commonly cited statistic in the tech industry, adding some credibility. Overall, while the article makes bold claims, it lacks direct citations to sources, which affects its verifiability.
The article primarily focuses on the optimistic potential of AI in the pharmaceutical industry, highlighting various benefits such as faster clinical trials and reduced drug development costs. However, it briefly acknowledges the challenges, such as the high failure rate of AI projects and the need for robust data governance. This creates a somewhat balanced view but leans heavily towards the positive aspects without deeply exploring the potential downsides or ethical concerns associated with AI in healthcare. Including more perspectives, such as those of skeptics or experts in data ethics, could have provided a more rounded discussion.
The article is generally clear and well-structured, with a logical flow from the introduction of AI's potential in the pharmaceutical industry to the challenges and solutions proposed. The language is accessible and avoids overly technical jargon, making it understandable to a broad audience. The use of bullet points for listing specific examples of AI applications helps in breaking down complex information and enhances readability. However, the clarity could be improved by providing more detailed explanations of certain terms, such as 'AI-ready data,' for readers unfamiliar with the topic.
The article lacks explicit citations to external sources, which makes it difficult to assess the reliability and credibility of the information presented. While it references well-known companies and entities like Gartner, Scilife, and OpenAI, the absence of direct quotes or links to studies or reports weakens the source quality. For a topic as complex and rapidly evolving as AI in pharmaceuticals, robust sourcing from industry experts, peer-reviewed studies, and official reports is crucial for maintaining credibility.
The article does not provide much transparency regarding the sources of its data or the methodology behind the claims. There is no disclosure of potential conflicts of interest, such as the author's affiliation with the industry or the publication's relationship with the companies mentioned. The lack of transparency about how projections and statistics were derived leaves readers without a clear understanding of the basis for these claims, which is essential for assessing the reliability of the information.
Sources
YOU MAY BE INTERESTED IN
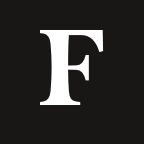
Digital Transformation In Sports: What’s In, What’s Out
Score 6.4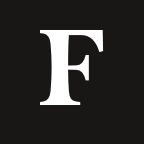
A Scientific, Economic And Ethical Reflection On Women's (Health) History
Score 6.2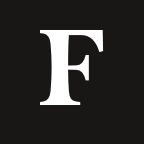