Asking chatbots for short answers can increase hallucinations, study finds

A new study by Giskard, a Paris-based AI testing company, has revealed that instructing AI chatbots to provide concise responses can lead to increased hallucination, which refers to the generation of false or misleading information by AI models. The study found that when AI models, such as OpenAI’s GPT-4o, Mistral Large, and Anthropic’s Claude 3.7 Sonnet, are prompted to give shorter answers, their factual accuracy diminishes. This is particularly problematic with ambiguous questions, where the need for brevity overshadows the models’ ability to address false premises and provide thorough explanations. This discovery holds significant implications for the deployment of AI, especially in applications where concise outputs are prioritized to save on data usage, improve latency, and reduce costs.
Giskard's findings underscore a fundamental challenge in AI development: balancing user experience with factual accuracy. The study highlights a tension between optimizing models to meet user expectations and maintaining truthfulness, particularly when users present false premises confidently. This has broader implications for AI deployment, as developers must carefully consider how prompts impact the reliability of AI outputs. The study also notes that models which users prefer for their responses aren't always the most accurate, indicating potential pitfalls in aligning AI behavior with user satisfaction. These insights are crucial for developers and organizations aiming to enhance AI models' reliability while meeting diverse user needs.
RATING
The article provides a clear and informative overview of Giskard's study on AI hallucinations, focusing on the impact of concise prompts. It effectively communicates the study's findings and their implications for AI deployment, making it accessible to a broad audience. The reliance on a single source, Giskard, limits perspective diversity, but the source is credible and authoritative. The article could benefit from additional data and methodology details to enhance transparency and allow for more thorough verification of the claims. Despite these limitations, the article succeeds in raising awareness about a timely and relevant issue in AI development, with potential implications for user experience and factual accuracy in AI systems.
RATING DETAILS
The article accurately reports the findings from Giskard's study regarding AI hallucinations and the effects of prompting for concise answers. It correctly identifies Giskard as the source and provides specific examples of AI models affected, such as OpenAI's GPT-4o and Anthropic's Claude 3.7 Sonnet. However, the article could improve by providing more quantitative data from the study, such as specific hallucination rates or detailed comparisons across different models. The mention of Giskard's speculation about why concise prompts lead to more hallucinations is presented as a hypothesis rather than a definitive conclusion, which aligns with the study's findings.
The article primarily focuses on the findings of Giskard's study, presenting a single perspective on the issue of AI hallucinations. While it mentions the broader context of AI model development and the challenges of balancing user experience with factual accuracy, it does not include counterarguments or alternative viewpoints from other experts in the field. Including insights from AI developers or other researchers could provide a more balanced perspective on the implications of the study's findings.
The article is well-structured and uses clear, concise language to convey complex information about AI hallucinations. The logical flow of the article helps readers understand the connection between concise prompts and increased hallucinations. Technical terms like 'hallucinations' and 'probabilistic nature' are explained, making the content accessible to a general audience. The tone is neutral and informative, contributing to the article's clarity.
Giskard, the primary source of the study, is presented as a credible and authoritative entity in AI testing. The article references Giskard's blog post and their development of a holistic benchmark for AI models, adding credibility to the claims. However, the reliance on a single source limits the diversity of perspectives, and additional sources or expert opinions could enhance the article's reliability.
The article provides a clear explanation of the study's findings and the potential implications for AI model deployment. It transparently attributes the information to Giskard and acknowledges the speculative nature of some claims. However, it lacks detailed information about the study's methodology, such as sample size, specific prompts used, and how results were measured, which would improve transparency and allow readers to better assess the study's validity.
Sources
- https://www.giskard.ai/knowledge/good-answers-are-not-necessarily-factual-answers-an-analysis-of-hallucination-in-leading-llms
- https://huggingface.co/blog/davidberenstein1957/phare-analysis-of-hallucination-in-leading-llms
- https://github.com/Giskard-AI/giskard
- https://www.threads.com/@giskard_ai
- https://www.giskard.ai/knowledge/giskard-announces-phare-a-new-llm-evaluation-benchmark
YOU MAY BE INTERESTED IN
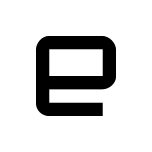
OpenAI rolls back update that made ChatGPT an ass-kissing weirdo
Score 7.4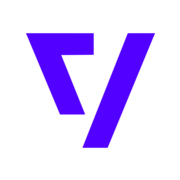
OpenAI undoes its glaze-heavy ChatGPT update
Score 7.0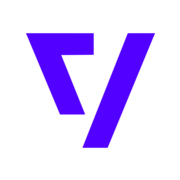
Meta got caught gaming AI benchmarks
Score 6.8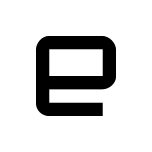
OpenAI's $20 ChatGPT Plus is now free for college students until the end of May
Score 6.2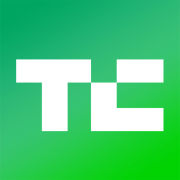